In the fabric of modern society, where the threads of morality, legality, and social norms are intricately woven together, the decision to seek the company of an escort often remains shrouded in secrecy. This discretion, while multifaceted in its origins, speaks volumes about the societal, personal, and professional landscapes that individuals navigate. This article delves into the reasons behind the veil of secrecy that surrounds the engagement with escorts, offering insight into the complex interplay of factors that drive individuals to maintain discretion about these encounters.
Societal Stigma and Moral Judgments
At the heart of the discretion surrounding the use of escort services lies the pervasive influence of societal stigma and moral judgments. Despite the progressive strides in many areas of social and sexual freedom, the engagement with a Miami escort remains a topic fraught with controversy and moral debate. This stigma stems from deep-seated beliefs about sexuality, morality, and the sanctity of personal relationships, often influenced by cultural, religious, and ethical frameworks that vary significantly across different societies.
Individuals who seek the companionship of escorts often do so knowing that their actions may be perceived as deviating from societal norms and expectations. The fear of being judged, ostracized, or condemned by family, friends, and the wider community compels many to keep these interactions under wraps. This secrecy is not necessarily an admission of guilt or shame but a protective measure against the potential fallout from societal judgment.
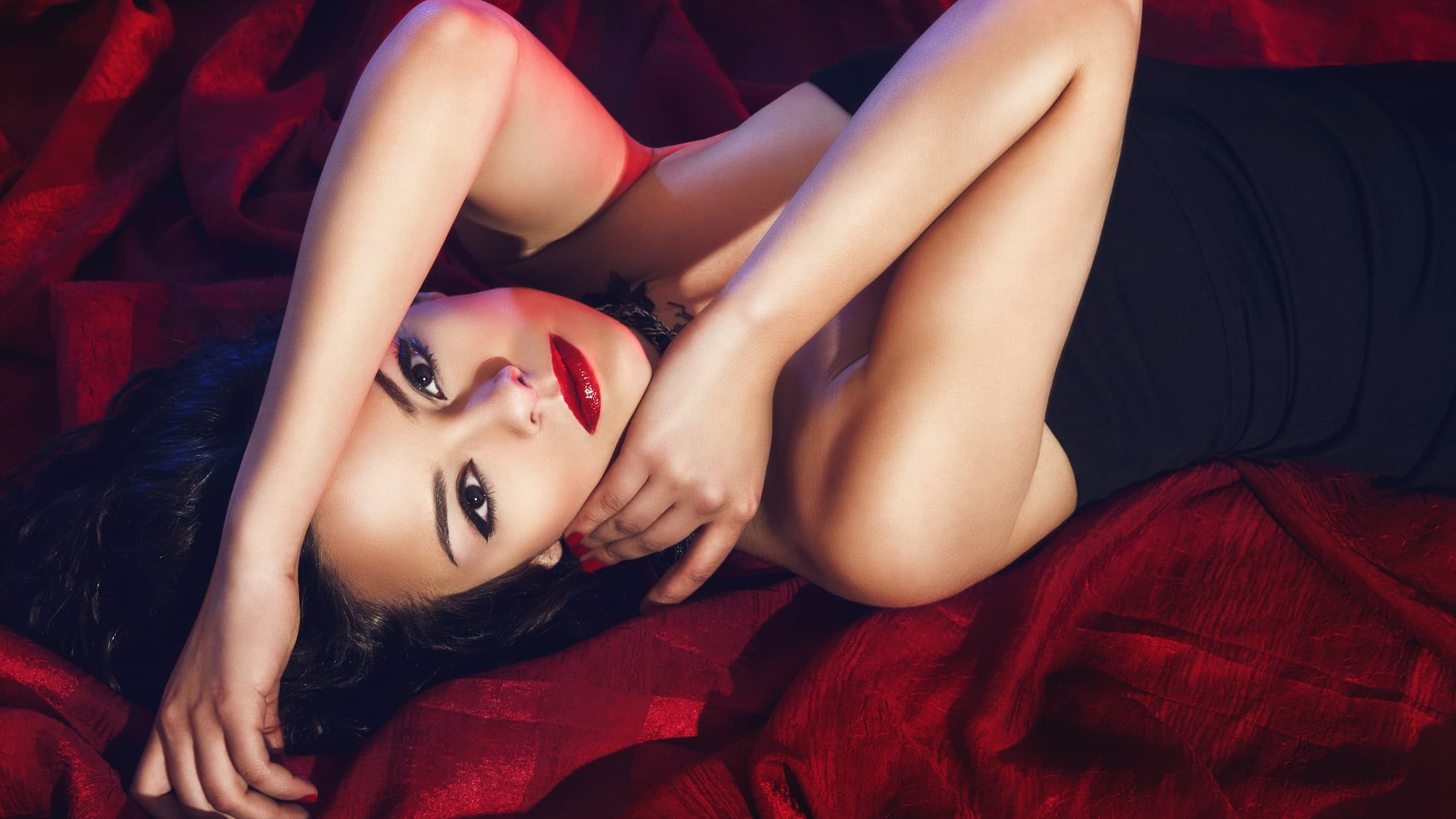
Professional Repercussions and Privacy Concerns
The decision to maintain secrecy about seeing escorts is also significantly influenced by considerations of professional reputation and privacy. In many professions, especially those held in high public or social regard, there is an implicit expectation of moral conduct that extends beyond the workplace. The revelation that an individual engages with escorts can lead to professional repercussions, including loss of job, damage to one’s reputation, and diminished prospects for future employment or advancement.
Moreover, in the digital age, where personal information is increasingly accessible and permanent, the stakes for privacy have never been higher. Individuals are acutely aware that details about their personal lives, once exposed, can be difficult to erase from the public domain. This concern for privacy extends to the fear of being blackmailed or exploited, further driving the need for discretion in their interactions with escorts.
The Pursuit of Authenticity and Uninhibited Expression
Beneath the layers of societal and professional considerations lies a deeply personal reason for secrecy: the pursuit of authenticity and uninhibited expression. For many, the interactions with escorts provide a rare space free from the expectations and judgments that permeate their daily lives. In the company of an escort, individuals can explore aspects of their identity, desires, and fantasies that they may feel compelled to suppress in other areas of their life.
This quest for authentic self-expression is a powerful motivator for discretion. The secrecy allows individuals to navigate the boundaries between their public persona and private desires, maintaining a compartmentalization that is both liberating and necessary for their sense of self. The fear that exposure could disrupt this delicate balance is a strong deterrent against sharing these experiences with others.
Conclusion
The reasons for maintaining secrecy about seeing escorts are as complex as the individuals who seek out their services. From navigating the treacherous waters of societal stigma and moral judgments to protecting one’s professional reputation and privacy, the layers of discretion are manifold. Beneath these external considerations lies a deeply personal quest for authenticity and uninhibited expression, further reinforcing the need for secrecy. Understanding these motivations requires a nuanced appreciation of the interplay between societal norms, personal identity, and the human desire for connection and exploration. As society continues to evolve, perhaps so too will the openness with which these interactions are discussed and understood, leading to a future where discretion is a choice rather than a necessity.